Self-driving car
A driver less car (also called autonomous car or self-driving car or self-driving vehicle, i.e. self-operating) is a vehicle that can perform certain tasks on its own, without the help of a human driver. The car uses sensors to analyze its environment, and doesn't need a human's help for certain tasks.[1] These tasks commonly include parking the car, driving while keeping the same lane on a highway or driving at a predefined speed. In public places, certain buses have been programmed to travel a given route. As of 2021, self-driving cars have been used in controlled environments more and more. An example of this might be buses that transfer passengers between airport terminals.

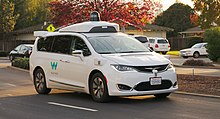
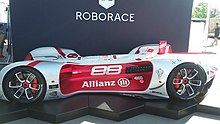
Technology
changeTypical technology used by a self-driving vehicle include Radar, lidar, digital cameras, machine learning, GPS and IMU.[2][3]
Cameras help the self-driving car detect objects to ensure safe navigation and enhance visibility to reduce accidents. Tesla, one of the self-driving cars has eight cameras around the car.[4] Three cameras provide a 120-degree field of view and are located in front behind the windshield. Two forward-looking side cameras and two rearward looking side cameras are on both sides of the car. One rear view camera at the back of the car. These cameras see changes in traffic signals, detect vehicles merging from adjacent lanes, and provide a wide range view.
LiDAR uses light waves to determine the distance from the car to other objects. It measures the time it takes for light to reflect off obstacles and return to the car.[5] Radar uses radio waves to detect the objects. Radar can measure the distance between car and objects and the speed of other moving objects.[6] LiDAR and Radar allow cars to understand more accurate surroundings. Radar and LiDAR technologies offer precise positional data, while camera excels at identifying objects. Combining these technologies significantly enhances the safety of self-driving cars, reducing the risk of accidents.
Machine learning technologies such as deep learning and reinforcement learning enable vehicles to recognize objects more accurately. For example, Tesla utilizes deep learning to train its vehicles in identifying various objects on the road, including pedestrians, lanes, and other vehicles.[7] This technology allows Tesla cars to continually improve their ability to navigate and respond to their surroundings, enhancing overall safety and performance. Reinforcement learning is utilized to help vehicles learn effective strategies for avoiding obstacles and other vehicles. Through repeated learning iterations, vehicles experience trial and error to learn how to navigate without errors.[8]
High-definition maps can be used to accurately locate a self-driving vehicle in a place, and to give information about parts of a road not seen by the car.[9]
Ethical issues
changeEven though it is technically possible to build cars that do certain things on their own, the question whether it should be done is different. Ethical questions include:
- Is a machine able to judge a situation correctly? – Can it make a difference between a stroller with a child, and a stroller with a doll?
- Is it acceptable to judge a situation by the expected death toll? (This has been formalized as the trolley problem.)
- If it is acceptable to judge such a situation by the expected death toll, what are meaningful criteria? – should the age of those killed be taken into account?
Legal issues
changeEven though these vehicles can already do a lot on their own, the laws of most countries still require that a driver is present. In many cases, self-driving buses also need a special permit from the government.
If a motor vehicle produces an accident, there always is the question of who is responsible, to determine what damages need to be paid. This also applies to self-driving vehicles: A self-driving vehicle is like a robot: Software is used for the driving. This software relies on a number of sensors to take decisions. Determining what went wrong in the case of an accident can be very difficult. This includes the question of who is legally responsible.
References
change- ↑ Gehrig, Stefan K.; Stein, Fridtjof J. (1999). Dead reckoning and cartography using stereo vision for an automated car. IEEE/RSJ International Conference on Intelligent Robots and Systems. Vol. 3. Kyongju. pp. 1507–1512. doi:10.1109/IROS.1999.811692. ISBN 0-7803-5184-3.
- ↑ Huval, Brody; Wang, Tao; Tandon, Sameep; Kiske, Jeff; Song, Will; Pazhayampallil, Joel (2015). "An Empirical Evaluation of Deep Learning on Highway Driving". arXiv:1504.01716 [cs.RO].
- ↑ Corke, Peter; Lobo, Jorge; Dias, Jorge (1 June 2007). "An Introduction to Inertial and Visual Sensing". The International Journal of Robotics Research. 26 (6): 519–535. CiteSeerX 10.1.1.93.5523. doi:10.1177/0278364907079279. S2CID 206499861.
- ↑ Tesla. (Nov 18, 2016). Advanced Sensor Coverage. https://www.tesla.com/autopilot
- ↑ Cadence System Analysis. (n.d.). The Use of RADAR Technology in Autonomous Vehicles. Cadence.
- ↑ Foresight. (Oct 27, 2022) Cameras, Radar, and Lidar: Which is the Right Choice for Autonomous Vehicles?. https://www.foresightauto.com/cameras-radar-and-lidar-which-is-the-right-choice-for-autonomous-vehicles/
- ↑ Tech&Tales. (Aug 28. 2023). How Tesla’s Self-Driving Cars Navigate the Road with Machine Learning. Medium. https://techntales.medium.com/how-teslas-self-driving-cars-navigate-the-road-with-machine-learning-b6f67052b8ba
- ↑ Tech&Tales. (Aug 28. 2023). How Tesla’s Self-Driving Cars Navigate the Road with Machine Learning. Medium. https://techntales.medium.com/how-teslas-self-driving-cars-navigate-the-road-with-machine-learning-b6f67052b8ba
- ↑ Liu, Rong; Wang, Jinling; Zhang, Bingqi (27 August 2019). "High Definition Map for Automated Driving: Overview and Analysis". Journal of Navigation. 73 (2): 324–341. doi:10.1017/S0373463319000638. ISSN 0373-4633. S2CID 202906063.